AI and machine learning models aid in retrosynthetic planning, but are limited by the training data they have seen. Read on to learn about ways to generate novel predictions by ensuring your data has the necessary diversity and quality to optimize key synthetic planning initiatives.
Evolution of existing drug molecules has been the innovation cornerstone of drug discovery. However, as we look to the future, structurally novel small molecules may prove to be more valuable therapeutics than adaptations of current drugs. With 65% of small molecule drugs approved in 2020 being structurally novel, these molecules are 2.5 times more likely to be designated as breakthrough therapies by the FDA, and 2 times as likely to become blockbuster drugs within 5 years of launch.
Synthesizing novel molecules, however, is no easy feat. Retrosynthetic prediction tools are becoming vital for the design of new approaches and optimization of production efficiency. These tools systematically leverage up-to-date research from across the globe to enable faster-to-market outcomes. Cost savings across the development pipeline can also be realized by building molecules with specific constraints, such as price or suppliers.
The successful application of AI to chemical synthesis is limited by data quality and diversity, the lack of which hinders prediction accuracy. This was demonstrated in our recent collaboration, where Bayer was seeking to optimize the use of AI for the retrosynthesis of novel small molecules. By enriching their existing training set with our high-quality, diverse reaction data, we were able to help improve the accuracy of the predictions being generated for rare reaction classes by 32 percentage points.
Diverse and accurate data drives AI model success
In chemical synthesis planning, the goal is to generate sets of synthetic routes that are as diverse and as accurate as possible. However, AI applications are only as good as the underlying data. The predictive power depends on the quality, diversity, and accuracy of the training data. A key challenge is data diversity, because if training data does not represent sparsely populated chemical subspaces, in addition to common chemistry, the AI application will deliver limited results in scope and novelty.
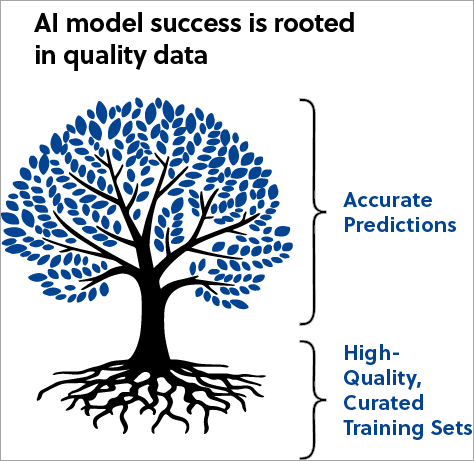
CAS Reactions offer a diverse range of reaction data which can significantly impact the predictive power of synthesis planning. The collection, more than doubling in size over the last ten years, curates the most robust and detailed chemical information from patents, journals, and scientific publications from across the world. This curation is ongoing and continues in tandem with machine learning to empower and enrich AI synthesis planning.
Bayer and CAS collaborate to maximize AI for more efficient drug discovery
In a collaboration between Bayer and CAS, a broad machine learning training set was enriched with CAS data targeting rare reaction types to dramatically enhance the predictive power of the drug discovery AI model.
The model comprised a viability filter consisting of a neural network that estimates whether a predicted reaction step is likely to be successful. The network was trained on a dataset of known reactions and a predominantly theoretical set of failed reactions. An additional training dataset was crafted with CAS data to quantify the predictive capability of the viability filter. Addition of the reactions from CAS increased accuracy in rare reaction classes from 16% to 48%, a boost of 32 percentage points.
Improvements in viability filter accuracy have a multiplying effect in the pipeline, creating a higher rate of usable reactions. This enhanced predictive power opens “rare” categories that previously eluded predictive models, contributing novel results that shine a light on the shaded areas of small molecule drug discovery.
This study demonstrates that even a moderately sized set of scientist-curated reactions from the CAS Content CollectionTM can significantly improve the predictive power of a synthesis planning tool. This effect was seen over just a small class of reactions, suggesting even greater predictive power would be seen with further augmentation of the base training set with strong, high-quality and diverse data across all templates. This impressive proof-of-concept has broad applications, most notably for more efficient discovery of novel small molecule drug targets.
- View the recent presentation by the Dr. Yugal Sharma, CAS, and Dr. Martin Villalba, Bayer, from the Pistoia Alliance Virtual Conference
- Download the whitepaper: Predicting new chemistry: The impact of high-quality training data on prediction of reaction outcomes
CAS can optimize your outcomes
CAS Custom ServicesSM can design training datasets to power your machine learning efforts. Contact our team to discuss your requirements and improve your predictive accuracy.